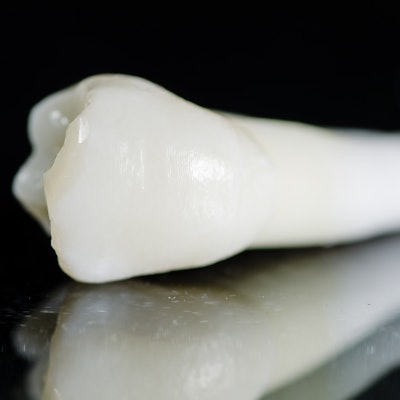
Combining cone-beam computed tomography (CBCT) and micro-CT images as training data can help artificial intelligence (AI) models identify the location of teeth and pulp cavities in CBCT images, according to a recent study published in the Journal of Endodontics.
The task, known as image segmentation, relies on large quantities of training data to locate objects of interest -- and their boundaries -- in images. The authors of the study proposed supplementing traditional manually annotated CBCT data, which may include features like the pulp cavity that are hard to identify, with data from micro-CT images.
Because CBCT training data are laborious to annotate, the group, led by Xiang Lin of the Tongji University Department of Endodontics in Shanghai, proposed combining the "advantages of high precision in micro-CT images and the clinical applicability of CBCT" (J Endod, September 11, 2021).
Specifically, the recommendation is to collect in vivo CBCT data and in vitro micro-CT data of the same tooth and then include the micro-CT data in the training samples for the CBCT image segmentation task. The new dataset may improve the accuracy of a previously developed AI model built for identifying tooth and pulp cavities on CBCT images, the authors wrote.
2 imaging methods
The team used two data pipelines to train their AI model: one pipeline contained images that had been manually labeled by an endodontic specialist, which was the control group; the other one contained images processed from the micro-CT data and was classified as the experimental group.
The researchers tested the two training methods using 30 single-root premolars, which were extracted for orthodontal purposes and collected between December 2019 and December 2020 from the Affiliated Stomatology Hospital at Tongji University. The teeth were imaged with a 3D Accuitomo CBCT machine and Scanco µCT 50 scanner.
To locate the pixel boundary of the teeth and pulp cavities, the team processed the CBCT data and created high-resolution CBCT images of each tooth.
For the control group, an endodontic specialist labeled the tooth and pulp cavity on the processed CBCT images using the open-source software Medical Imaging Interaction Toolkit (MITK).
For the experimental group, an endodontic specialist labeled the tooth masks (i.e., shadings that delineate the object's pixels from other objects or references in the image) on CBCT images. These masks were used to construct a 3D tooth model without a labeled pulp cavity.
Using micro-CT images, a technician then used segmentation to reconstruct a corresponding high-precision, micro-CT 3D model of each tooth that included the pulp cavity.
The two models were then aligned so the micro-CT model with the pulp cavity was positioned on the CBCT images without the pulp cavity. The researchers used the MITK software to map the highest precision data of the 3D micro-CT model on the 2D CBCT images.
More accurate pulp cavity models
Overall, the pulp cavity segmentation results from the experimental group were encouraging, the authors noted. The experimental group had a higher precision rate in the pulp chamber and a higher recall rate in the root canal, indicating that the algorithm trained by both CBCT and micro-CT samples achieved better pulp cavity segmentation.
"The method proposed in this study could make the process of obtaining the 3D tooth and pulp cavity models based on CBCT images more automated and accurate," the authors wrote. "This will greatly expand the application prospects of 3D models reconstructed from CBCT in the clinical diagnosis and treatment of endodontics."
In future experiments, the researchers suggested using teeth with more complex structures, such as molars. Because the study was restricted to isolated teeth, future work should focus on endodontic disease, such as root fractures and calcified root canals, the group noted.